UnAI-able™ Services & Solutions
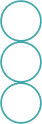
We make data and AI concepts snackable from the classroom to the boardroom. Filling knowledge gaps between data/AI fluency and AI adoption activities of an organization's workforce are where we excel. Our clients clarify their tech strategy to better align people, processes, practices and policies.
Our Process

Item #0
Step 1: Assessment

Begin with a preliminary questionnaire to understand the client’s data and responsible AI understanding, pain points and desired outcomes.
Step 2: Needs

Conduct a scope call to delve deeper into the client’s requirements and priorities, refining the roadmap for addressing identified data and responsible AI gaps.
Step 3: Design

Craft and tailor the data ethics literacy program. Iterate with client to ensure it meets the identified needs and aligns with the client’s objectives.
Step 4: Program Delivery

Request regular feedback to assess its effectiveness in enhancing understanding and delivering expected impact
Step 5: Feedback

Begin with a preliminary questionnaire to understand the client’s data and responsible AI understanding, pain points and desired outcomes.
Step 6: Recommendations & Collaboration
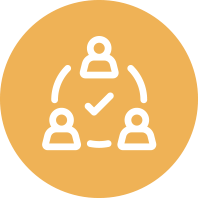
Based on program delivery and feedback engagement, generate a new set of recommendations and collaboratively discuss ongoing needs and how they can be addressed through further collaboration, ensuring continuous improvement and alignment with evolving objectives.

Item #0
Assessment

Begin with a preliminary questionnaire to understand the client’s data and responsible AI understanding, pain points and desired outcomes.
Needs

Conduct a scope call to delve deeper into the client’s requirements and priorities, refining the roadmap for addressing identified data and responsible AI gaps.
Design

Craft and tailor the data ethics literacy program. Iterate with client to ensure it meets the identified needs and aligns with the client’s objectives.
Program Delivery

Request regular feedback to assess its effectiveness in enhancing understanding and delivering expected impact.
Feedback

Begin with a preliminary questionnaire to understand the client’s data and responsible AI understanding, pain points and desired outcomes.
Collaboration
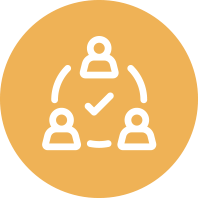
Based on program delivery and feedback engagement, generate a new set of recommendations and collaboratively discuss ongoing needs and how they can be addressed through further collaboration, ensuring continuous improvement and alignment with evolving objectives.
Case Studies
Case Study

Portland Community College AI Symposium
Unlocking the power of Artificial Intelligence in Education, Industry & Everyday Life.
Needs
- PCC identified the need to address AI’s significance and sought a keynote speaker for comprehensive education.
- The objective was to provide clear insights into AI’s definition, implications, and historical context.
- Effective communication to a diverse audience with varying familiarity levels was crucial for knowledge dissemination.
Delivery
- 120 Minute Keynote address that simplified complex AI concepts, making them accessible to all.
- Illustrated the evolution of AI with relatable examples and historical references.
- Audience deepened their understanding of the data-AI skills gap through engaging discussions and questions.
Outcomes
- Dr. Marshall’s keynote revolutionized AI perceptions and inspired ongoing initiatives at PCC.
- Attendees left enlightened and driven which resulted in continuing sparking transformative discussions.
- Dr. Brandeis’s book galvanized research and helped foster a culture of Responsible AI integration at PCC.
Case Study

General Services Administration Equity Study
Evaluating equity in remote identity proofing solutions
Needs
- GSA identified a need for a culturally competent data scholar to help them achieve their equitable access goals.
- The objective was to help the team interpret the vendor’s performance and identity verification capabilities.
- An external perspective on assessing the data gaps in the forgery and similarity fraud scenarios across pre-determined demographics.
Delivery
- Weekly meeting attendance to review and collaborative discuss newly process vendors’ results.
- Illustrated the considerations, pain points and implications between statistical errors and societal biases.
- Shared feedback, recommendations and resources centering data equity strategies.
Outcomes
- Dr. Marshall’s guidance helped standardize data collection and documentation processes.
- The GSA team was better equipped to distinguish amongst algorithmic errors, network errors and bias across demographic groups.
- Dr. Marshall and the GSA team are contributing to facial verification research through public scholarship dissemination.